Synthetic Data Is a Dangerous Teacher
Synthetic Data Is a Dangerous Teacher
Synthetic data, while it may seem harmless and convenient, can actually be a dangerous teacher. The use of synthetic data in training machine…
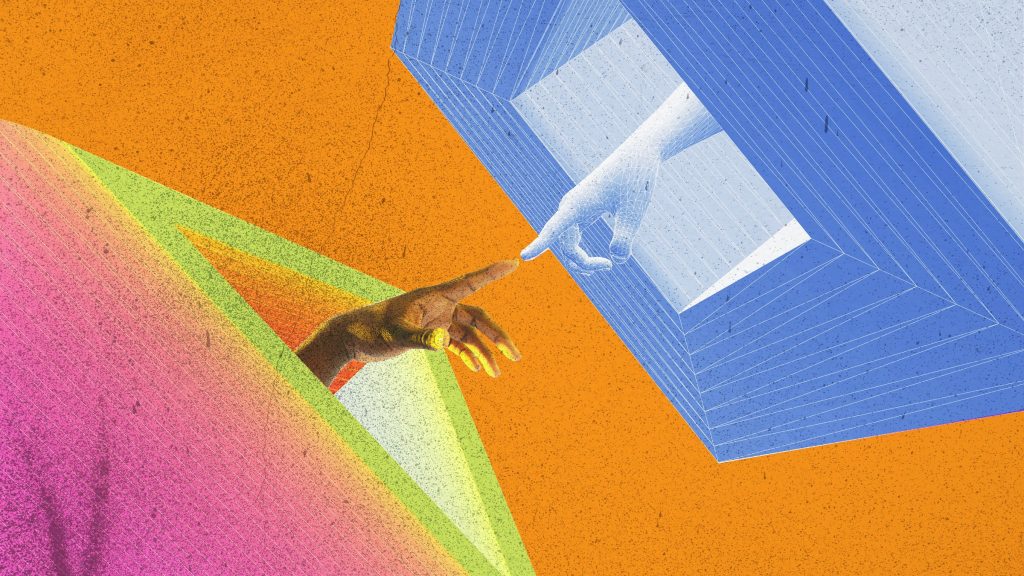
Synthetic Data Is a Dangerous Teacher
Synthetic data, while it may seem harmless and convenient, can actually be a dangerous teacher. The use of synthetic data in training machine learning models can lead to biased and inaccurate results. This is because synthetic data does not accurately reflect the complexities and nuances of real-world data.
One of the major issues with synthetic data is that it lacks the variability and nuances that real-world data possesses. This can lead to models that perform poorly in the real world, as they have not been trained on data that accurately reflects the complexity of the problem at hand. Additionally, synthetic data can introduce biases into models, leading to unfair or discriminatory outcomes.
Another danger of synthetic data is that it can give a false sense of security. Models trained on synthetic data may perform well in controlled environments or on test datasets, but fail when deployed in the real world. This can have serious consequences, especially in fields such as healthcare or finance where accurate predictions are crucial.
It is important for data scientists and machine learning engineers to be wary of the limitations of synthetic data and to use it judiciously. While synthetic data can be a useful tool for augmenting training datasets or generating new data points, it should not be relied upon as a substitute for real-world data. By understanding the dangers of synthetic data, we can work towards building more robust and accurate machine learning models.
In conclusion, synthetic data may seem like a harmless shortcut, but it can actually be a dangerous teacher. It is crucial for those working with machine learning models to be aware of the limitations of synthetic data and to use it responsibly. Only by incorporating real-world data and understanding the complexities of the problem at hand can we ensure that our models are truly effective and unbiased.